Data Warehouse: How Does a Data Warehouse Work?
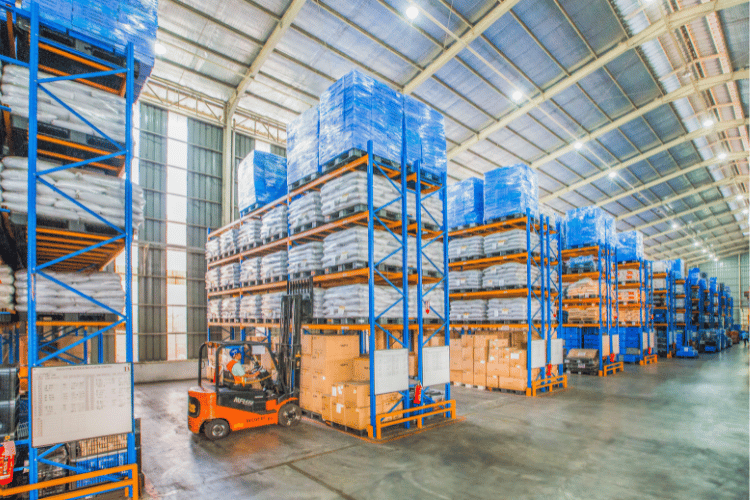
Data warehouse: what is it? How does a data warehouse work? What is the architecture of a DWH? On this page, you will find all the answers.
Companies need to understand their data and design a business intelligence project to enable digital transformation. Data warehouses are easy to create, but choosing a data warehouse architecture is a complex process.
How do traditional data warehouse models work, and what are the challenges of using the cloud?
A Data Warehouse (DWH): What Is It?
A data warehouse is a database that collects, stores, and analyzes large amounts of data from various sources.
The goal is to organize and structure this unstructured material to extract useful information and make it accessible. Bill Inmon and Ralph Kimball popularized this theory in the 1990s. As a result, decision support systems use operational data to make decisions.
The correct data warehouse architecture is a solid foundation for business intelligence solutions at a time when organizations are struggling to store and use data.
Recent analysis predicts that the data warehouse market, valued at $21 billion in 2020, will grow to $34 billion by 2025.
Depending on your business goals, you must choose between a data warehouse and a data lake.
Three Tiers in A Traditional Data Warehouse Architecture
A three-tier architecture, sometimes referred to as a three-tier or three-tier architecture, is the foundation of a traditional data warehouse.
Tier 1: Access
The database server is part of the first tier. It retrieves information from a variety of sources, including transactional databases.
Tier 2: Processing
Business rules and application logic are implemented by the Online Analytical Processing (OLAP) server, which is part of the second tier. Here, a structure is created from the data that can be used for queries and analysis.
Tier 3: Demonstration
The client layer, the area the user sees and interacts with, is the final third. This is where the data reporting, retrieval, and analysis functions reside.
Models In the Context of Traditional Data Warehouse Concepts
In a typical project, there are three different types of data warehouses.
1. Virtual Data Warehouse (VDW)
Individual databases that can be accessed simultaneously are called virtual data warehouses (VDW). This allows the user to access all the data as if it were in one data store.
2. Data Mart
Data marts emerged in the 1970s to facilitate access to company, application, or domain data. For example, commercial or financial data marts consist of specific data that solves a functional problem. Data marts are topic-specific and have limited coverage, while data warehouses are broader and cover more topics.
3 Enterprise Data Warehouse
Paul Murphy and Barry Devlin of IBM created the Enterprise Data Warehouse (EDW), which emerged in the late 1980s. These centralized data warehouses housed enterprise-wide data. They allowed companies to maximize their information resources and categorize data into specific topics.
Methods For Entering Data into A Data Warehouse
An extract-transform-load (ETL) method is used to extract information into a data warehouse. The purpose of this type of software is to collect, organize, and transform data into a format that the data warehouse can use. Once loaded, the structured data can be categorized.
New Cloud Data Warehouse Architectures
Organizations are moving their databases and data warehouse tools to the cloud along with their operations.
On-demand cloud data warehousing has grown rapidly in recent years. Managed services such as Google BigQuery, Snowflake, Microsoft Azure SQL Data Warehouse, and Amazon Redshift offer simple and efficient solutions.
The Advantages of Such Cloud Data Warehousing (CDW) Are Many:
1. Greater ease of use,
2. Less version control obligations,
3. Faster and more efficient development compared to on-premises development,
4. Faster, easier, and cheaper deployment; Better risk and cost management.
However, they do not conform to traditional architecture.
Each data warehouse has its architecture, which creates a number of challenges. These include high processing load, dealing with semi-structured data, lack of nested structures, constant cluster reconciliation, query optimization, and routine maintenance for data backup and recovery.
Do you have questions? Contact experienced data warehouse consulting experts to discuss BI and data warehouse architecture.
- Art
- Causes
- Crafts
- Dance
- Drinks
- Film
- Fitness
- Food
- Games
- Gardening
- Health
- Home
- Literature
- Music
- Networking
- Other
- Party
- Religion
- Shopping
- Sports
- Theater
- Wellness